통계/time series
조금 더 빠른 시계열 예측
cj92
2020. 6. 8. 22:29
시계열 예측 관련 공부를 하며 walking forward validation을 사용하는 경우가 발생하게 되었는데, 기존 데이터가 너무 커서 시간이 너무 오래걸리는 문제를 느꼈다.
그래서 과연 어떻게 하면 빨리 모델링을 할 수있을까? 확인해보고자 했다.
forecast 패키지를 활용하여 성능 비교를 해보자.
먼저 사용된 패키지는 data.table, zoo, forecast를 활용하였다.
time3=system.time({
ls=list()
for(i in 1:25){
message(i)
ls[[i]]=data.frame(forecast(auto.arima(AirPassengers[1:(120+i-1)],ic='aicc',stepwise=F),12))[,1]
temp3=t(bind_cols(ls))
}
})
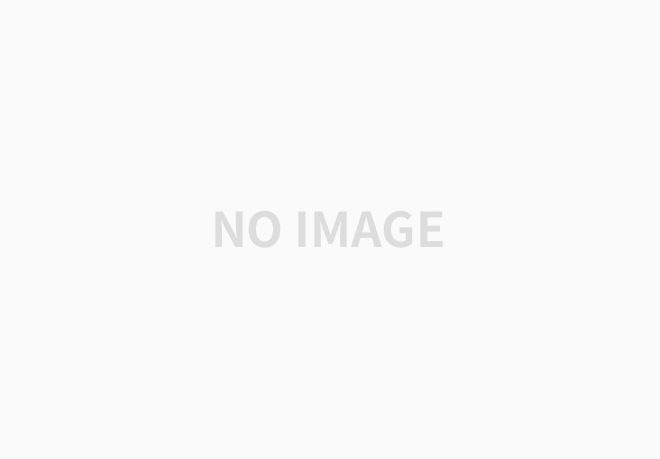
먼저 for문을 통해 시간을 계산 한 결과 11초 정도가 나타났다.
다음은 lapply문을 활용한 결과 큰 차이는 나지 않으나 조금 빨라진 모습을 볼 수 있다.
time4=system.time({
ls2=list()
for(i in 1:25){
ls2[[i]]=AirPassengers[1:(120+i-1)]
}
temp4=lapply(ls2,function(x){data.frame(forecast(auto.arima(x,ic='aicc',stepwise=F),12))[,1]})
})
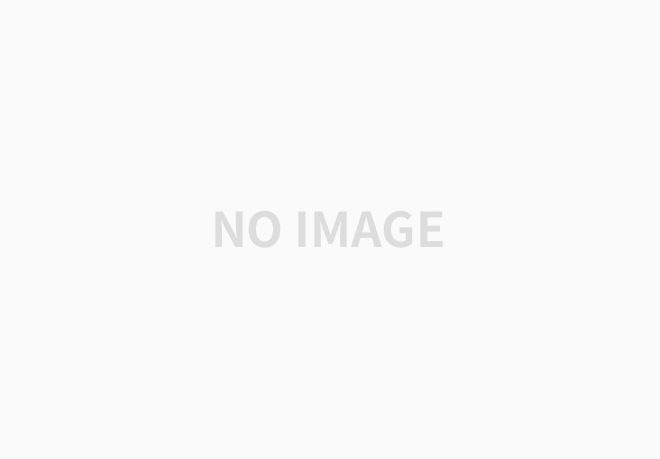
다음은 zoo 패키지의 rollapply를 사용한 결과이다. 조금 더 빨라진 모습을 볼 수 있다.
library(forecast)
time1=system.time({
dt=data.frame(temp=AirPassengers)
setDT(dt)
temp1=dt[,rollapply(temp,120,function(x){data.frame(forecast(auto.arima(x,ic='aicc',stepwise=F),12))[,1]})]
})
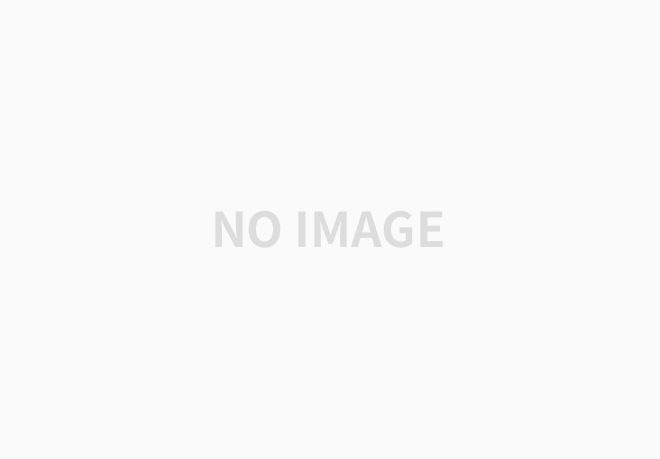
다음은 data.table 패키지를 활용한 결과이다.
time1=system.time({
dt=data.frame(temp=AirPassengers)
setDT(dt)
temp1=dt[,rollapply(temp,120,function(x){data.frame(forecast(auto.arima(x,ic='aicc',stepwise=F),12))[,1]})]
})
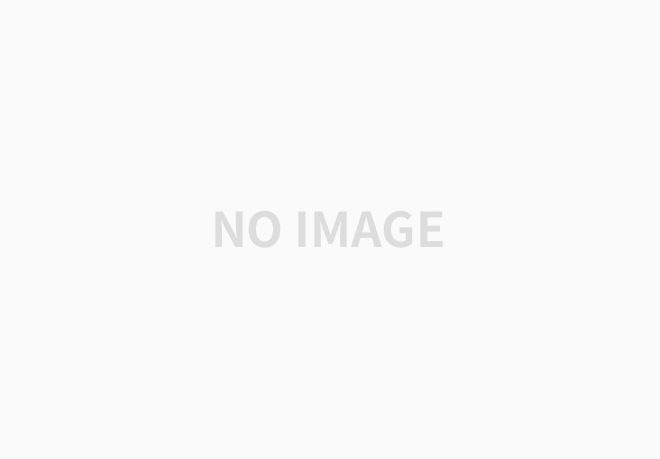
끝으로 아래 포스팅에서 본 여러개의 시계열 모델을 빠르게 예측하는 방법을 올리고 포스팅을 마치겠다.
https://statkclee.github.io/statistics/stat-time-series-forecast.html
Software Carpentry: 데이터 과학 – 기초 통계
데이터 과학 – 기초 통계 시계열 데이터 예측(forecast) 학습 목표 시계열 데이터의 백미 예측을 살펴본다. 다양한 시계열 데이터 모형의 장단점을 비교한다. 자동 시계열 예측의 필요성을 이해한
statkclee.github.io